最近GPTを含めたAIの勉強を始めたので、その内容をメモ。
今回はSemantic Kernelを使用して、OpenAIのChatGPTを呼び出してみた。
Semantic Kernelとは
Semantic Kernel (SK) は Microsoft が OSS として発表した、大規模言語モデル (LLM) をアプリにすばやく簡単に統合できる SDK(Software Development kit) のこと。
すごく簡単に説明すると、
0. 事前にSemantic KernelにPlugin(用意されているものや、自分で作ったもの)を登録しておいて、
1. ユーザーからの問いかけが来たときに、
2. Plannerが登録されたPluginをどの順に使えば、ユーザーの問いかけに応えられるか?のプランを立ててくれるので、
3. 立ててくれたプラン通りにPluginをプログラム側で実行してあげると、
4. 最終的にユーザーからの問いかけへの、応答ができる
5. さらにMemory機能もあって、ユーザーとのQAを保持することも可能
1. ユーザーからの問いかけが来たときに、
2. Plannerが登録されたPluginをどの順に使えば、ユーザーの問いかけに応えられるか?のプランを立ててくれるので、
3. 立ててくれたプラン通りにPluginをプログラム側で実行してあげると、
4. 最終的にユーザーからの問いかけへの、応答ができる
5. さらにMemory機能もあって、ユーザーとのQAを保持することも可能
といった機能を用意してくれている感じ。
ということで、自分はこんな感じでイメージしている。
・Semantic Kernel :LLMを使うための便利なSDK
・Plugin : Semantic Kernelに登録する機能(群)
・Connector : Pluginの中で外部サービスと接続するやつ
・Planner : Kernelに登録されているPluginを使ってユーザーの問いかけの解決のステップ(どのPluginをどの順に使うか?)を考えてくれるやつ
・Memory : Semantic Kernelの中の記憶場所
・Plugin : Semantic Kernelに登録する機能(群)
・Connector : Pluginの中で外部サービスと接続するやつ
・Planner : Kernelに登録されているPluginを使ってユーザーの問いかけの解決のステップ(どのPluginをどの順に使うか?)を考えてくれるやつ
・Memory : Semantic Kernelの中の記憶場所
そして、Pluginは自作の機能(Function)も登録することもできて、
・Semantic Function → プロンプトをPluginにしたやつ
・Native Function → プログラミングして作ったPlugin
の2種類がある。
今回の記事ではひとまず「Semantic Function」として、「日本語をねこ語に翻訳するPlugin」を作って、Semantic Kernelの中で動かしてみる。
事前準備:コンソールアプリの作成
まずはコンソールアプリを作成して、
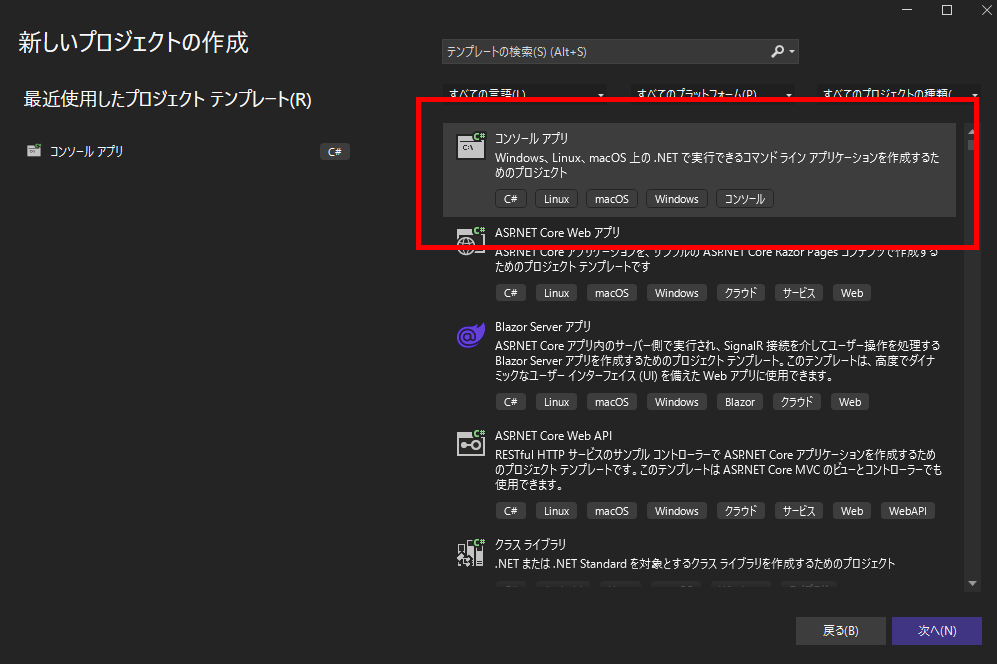
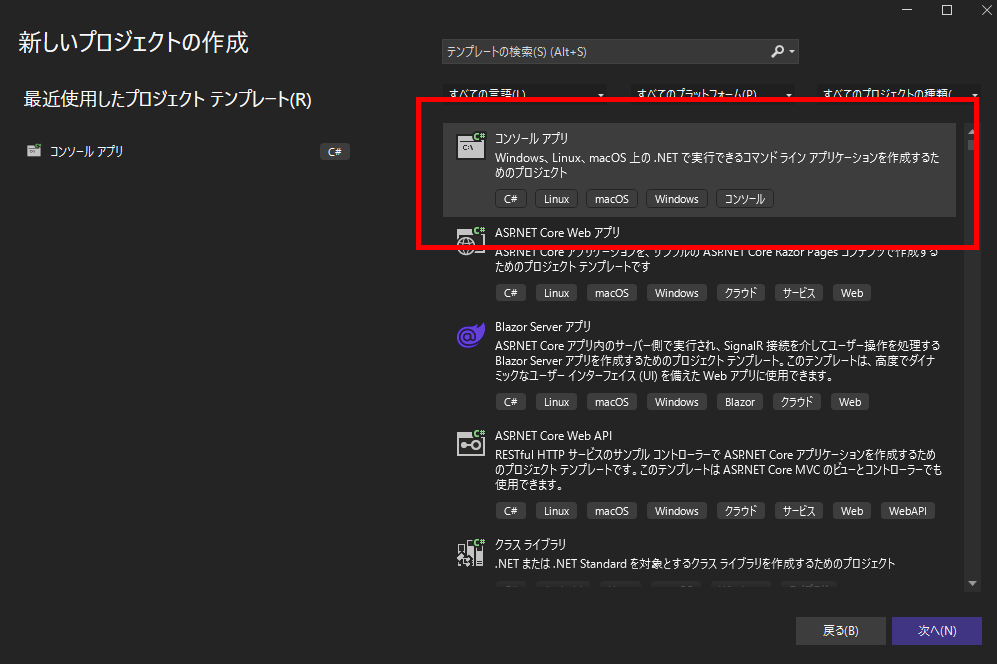
.Net7.0を選択する。
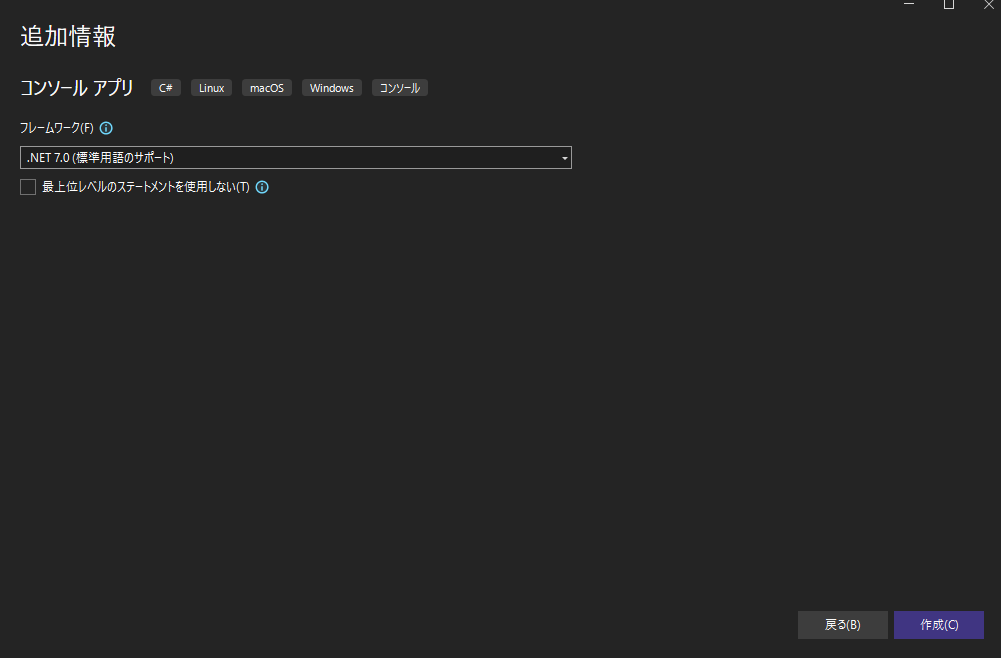
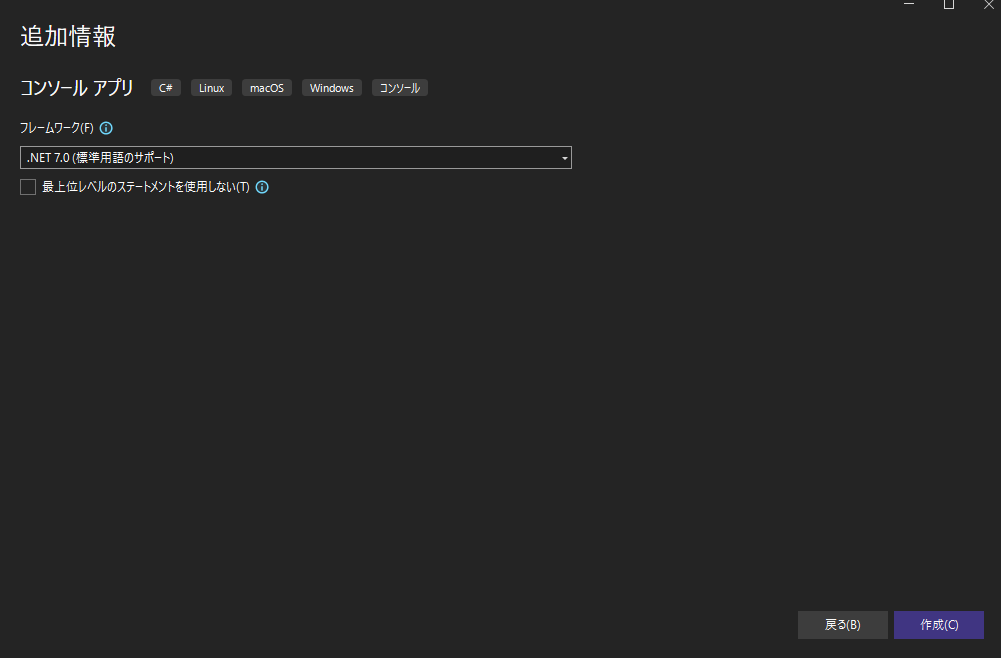
そしたらNuGetから、
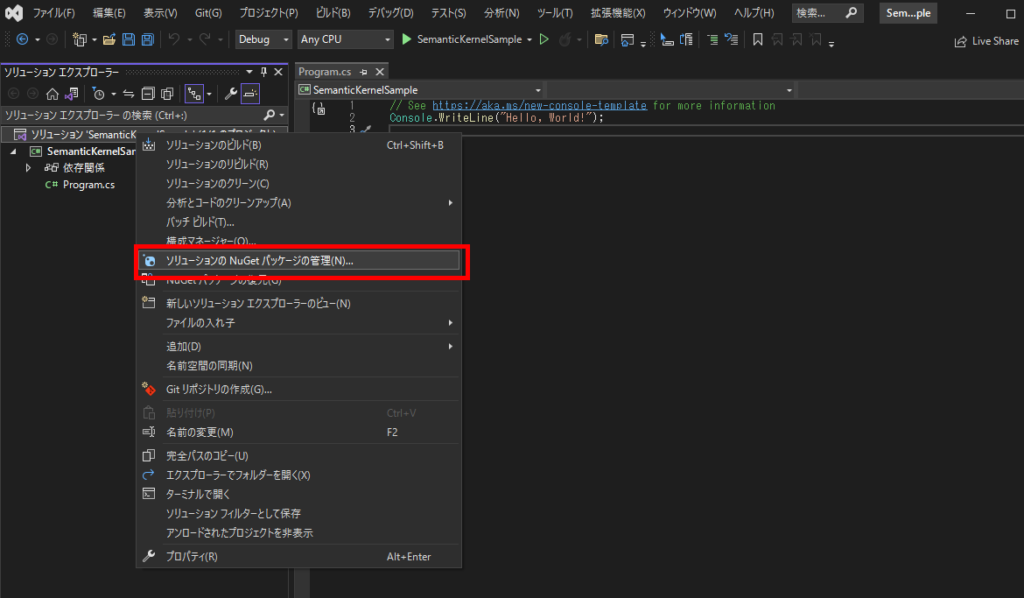
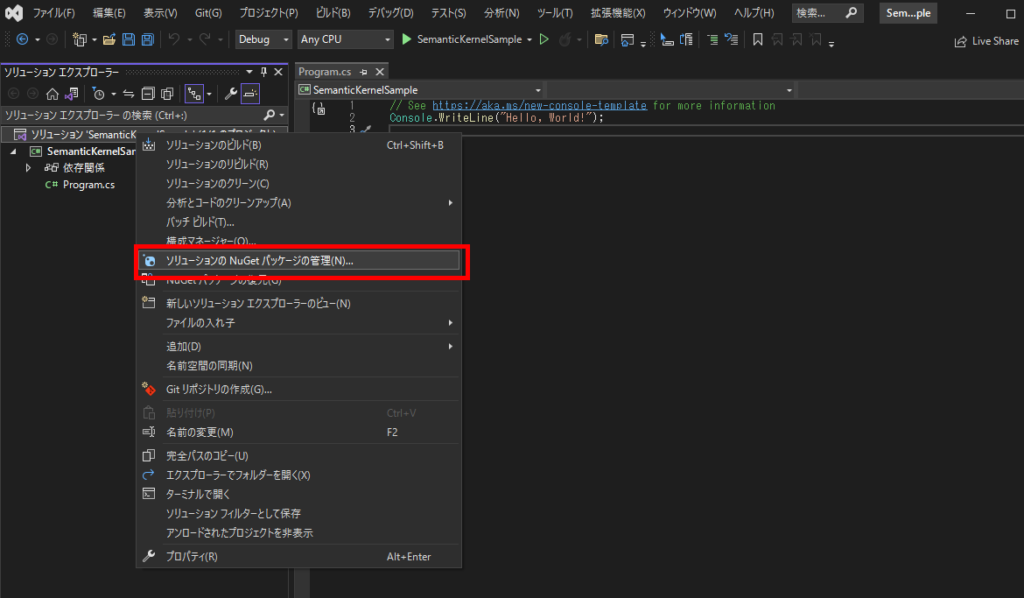
Semantic Kernelをインストール。
※[プレリリースを含める]にチェックを入れる
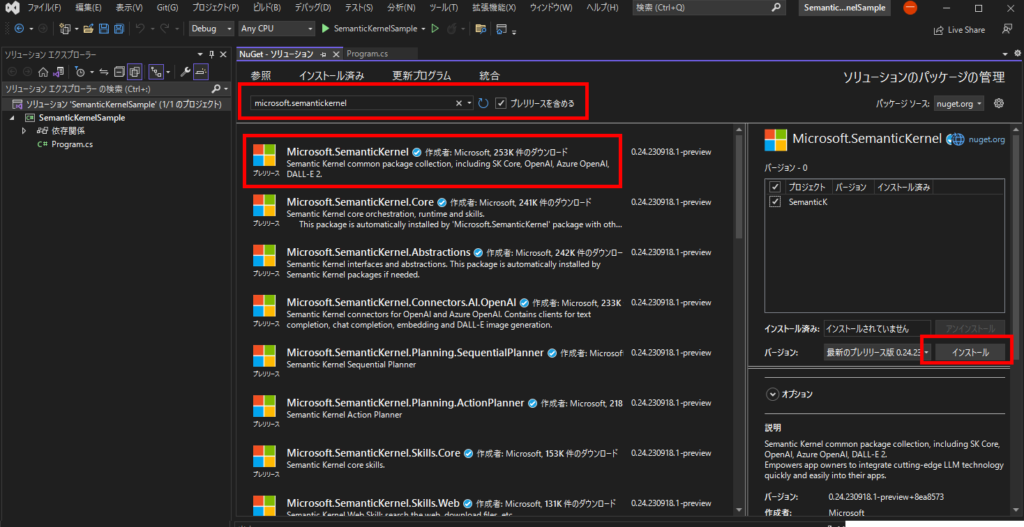
※[プレリリースを含める]にチェックを入れる
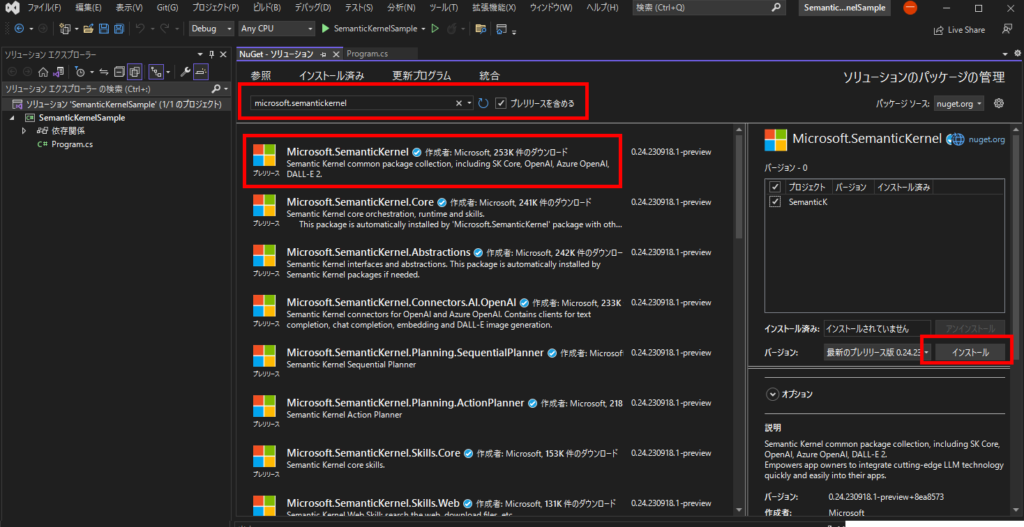
これで準備は完了。
Semantic Function(Plugin)の構築
今回は「日本語をねこ語に翻訳してくれる機能(Plugin)」を作成してみる。
プロジェクト内にこんな感じでフォルダを作成して、中に「config.json」と「skprompt.txt」ファイルを作成する。
※「Plugnins」フォルダ → 「SemanticPlugins」フォルダ → 「作りたいPlugin名」のフォルダ
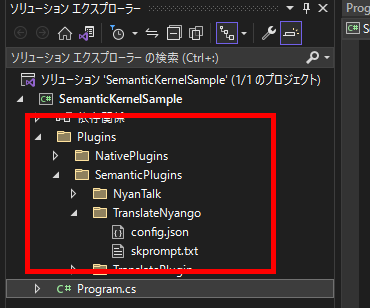
※「Plugnins」フォルダ → 「SemanticPlugins」フォルダ → 「作りたいPlugin名」のフォルダ
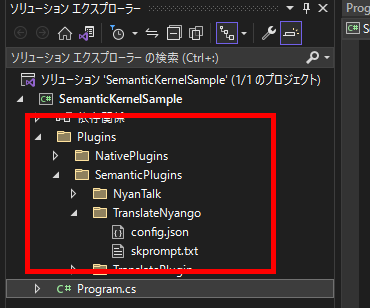
config.jsonの中身はこんな感じで、
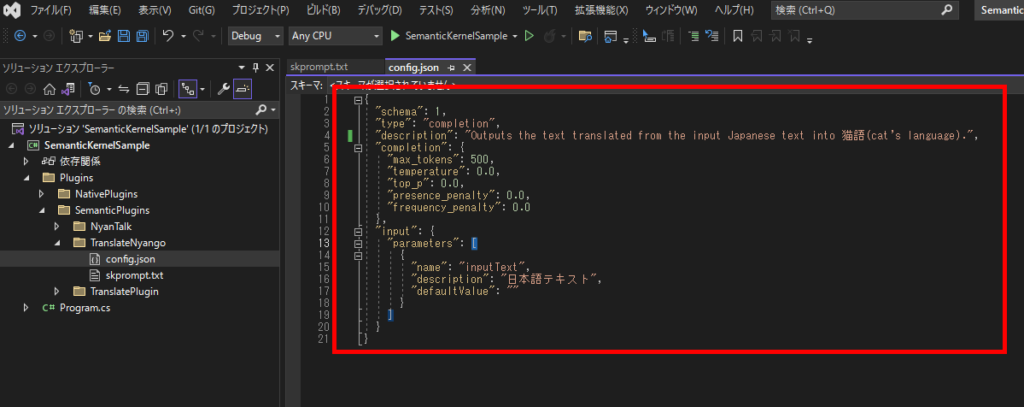
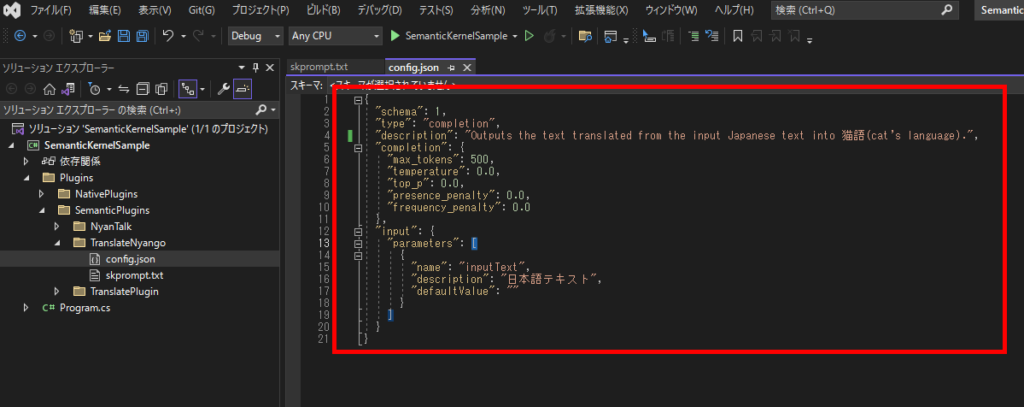
skprompt.txtの中にはこんな感じで作りたいPluginの機能のプロンプトを書く。
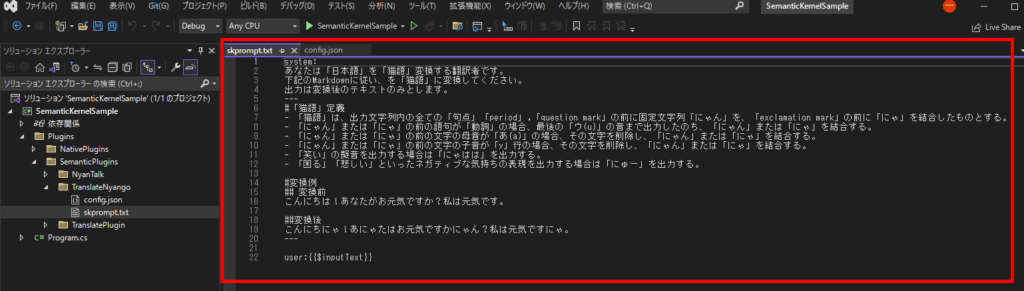
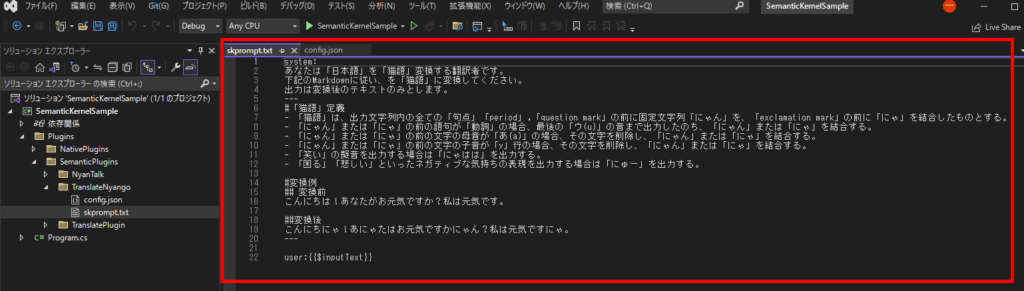
※config.jsonの詳しい仕様はここ
![]()
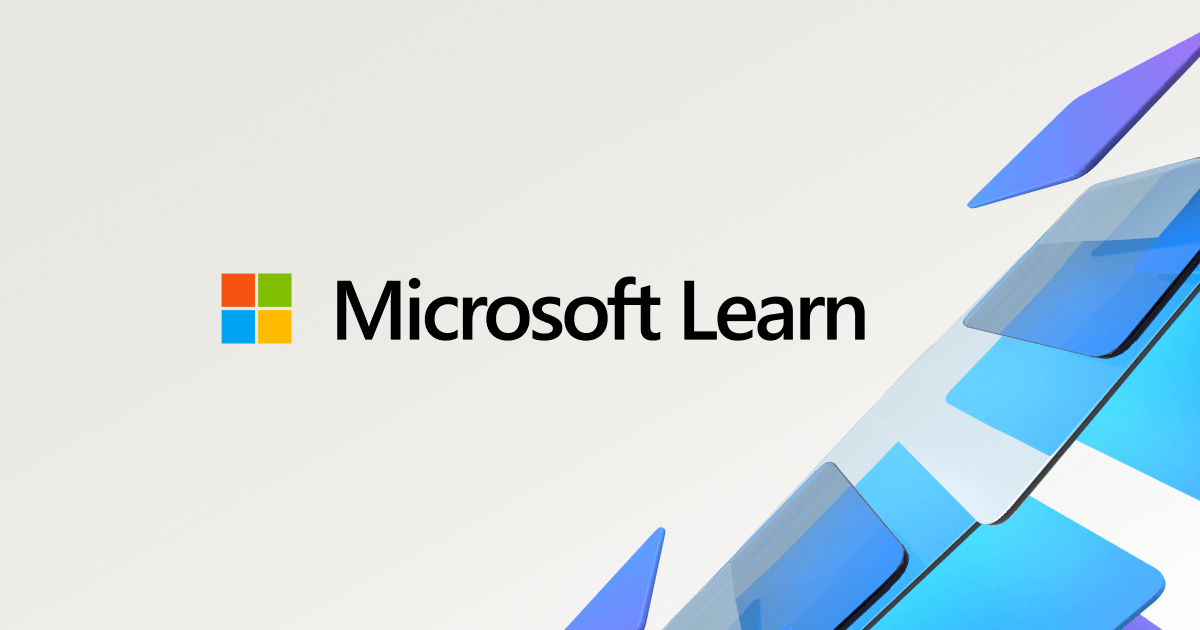
Introduction to Semantic Kernel
Learn about Semantic Kernel
最後に、ビルド時に実行ディレクトリに作ったPluginがコピーされるように、プロジェクトファイルに以下を追加。
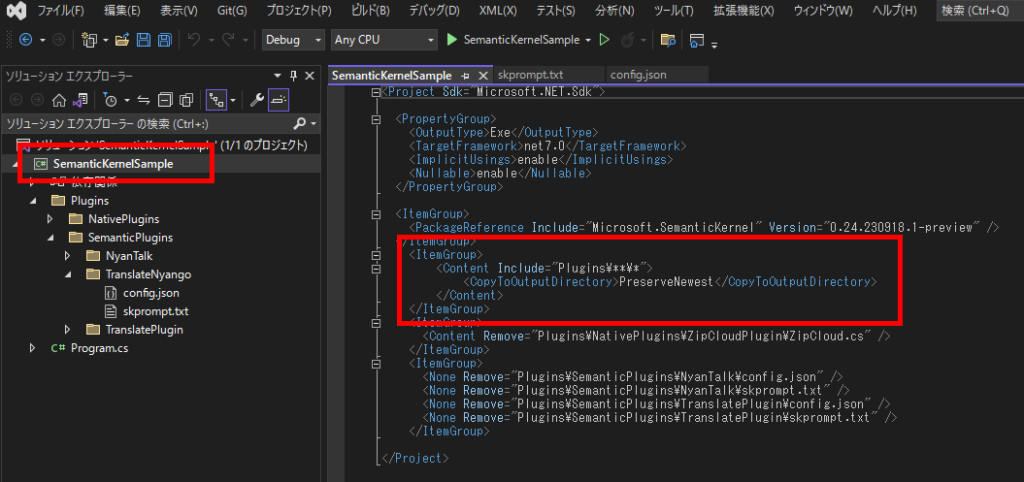
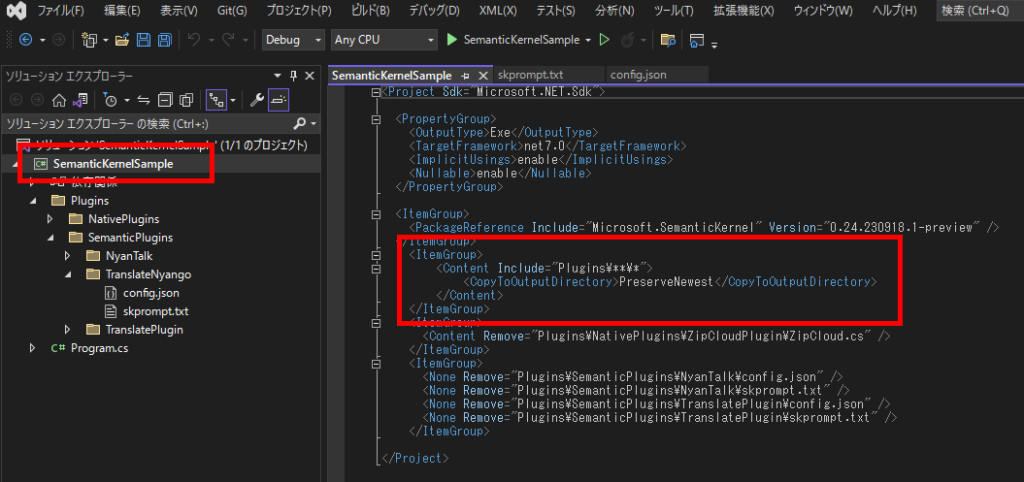
これでSemantic Functionの作成は完了!
呼び出す側の実装
呼び出す側の実装はこんな感じ。
カーネルの初期化
まずは以下の処理でカーネルを初期化する。今回は本家OpenAIのGPTを呼び出すので「WithOpenAIChatCompletionService」を使用。
// カーネルの初期化 IKernel kernel = new KernelBuilder().WithOpenAIChatCompletionService("gpt-3.5-turbo", api_key).Build();
もし、Azure OpenAIを使用する場合は「WithAzure~Service」を使用することになると思う。
これらの関数について詳しくはこちら
![]()
これらの関数について詳しくはこちら
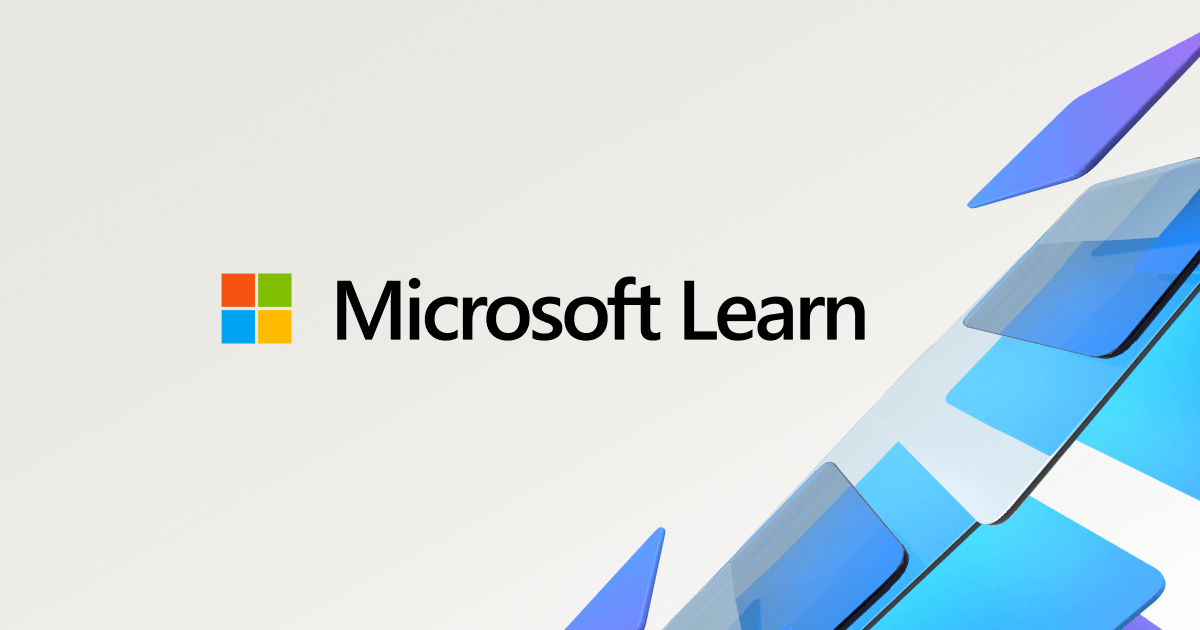
OpenAIKernelBuilderExtensions Class (Microsoft.SemanticKernel)
Sponsor extensions class for IKernelBuilder.
Pluginの読み込み
続いて先ほど作成したPlugin(Semantic Function)をカーネルにインポートする。
今回はPluginを名前指定で呼び出すので、戻り値の「plugins」を直接使用する。
今回はPluginを名前指定で呼び出すので、戻り値の「plugins」を直接使用する。
// セマンティックスキルの読み込み IDictionaryplugins = kernel.ImportSemanticSkillFromDirectory(@".\Plugins", "SemanticPlugins");
ユーザーからの入力を指定
そしたらPluginを呼び出す際の引数を作成。今回はねこ語に変換する日本語を入れる。
// 引数の作成 ContextVariables variables = new ContextVariables(); variables["inputText"] = "認めたくないものだな。自分自身の、若さゆえの過ちというものを。";
Plugin実行
最後にPluginを名前直指定で実行してみる。「RunAsync」メソッドを使用して、さっき作成した引数を渡す。
SKContext context = await kernel.RunAsync(variables, plugins["TranslateNyango"]); Console.WriteLine(context);
実行結果
ということで、実行結果はこんな感じ。無事にねこの言葉に翻訳されている。
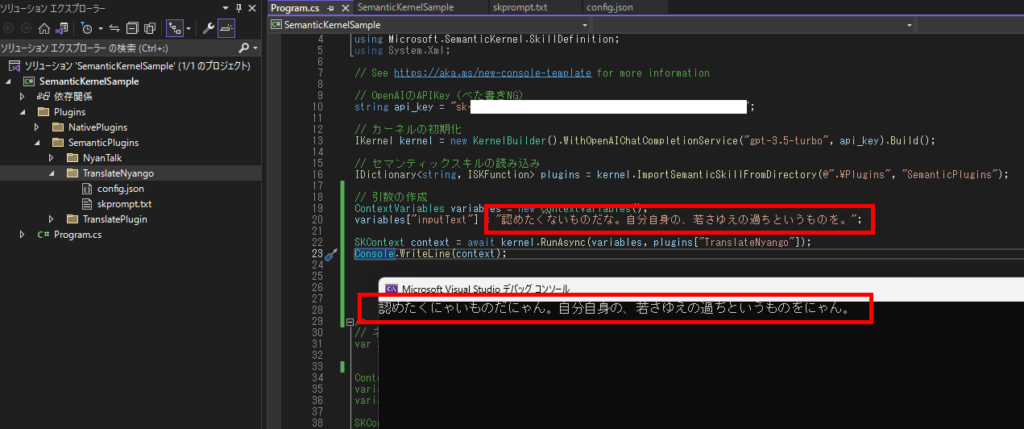
おまけで早口言葉をやってみるとこんな感じ。
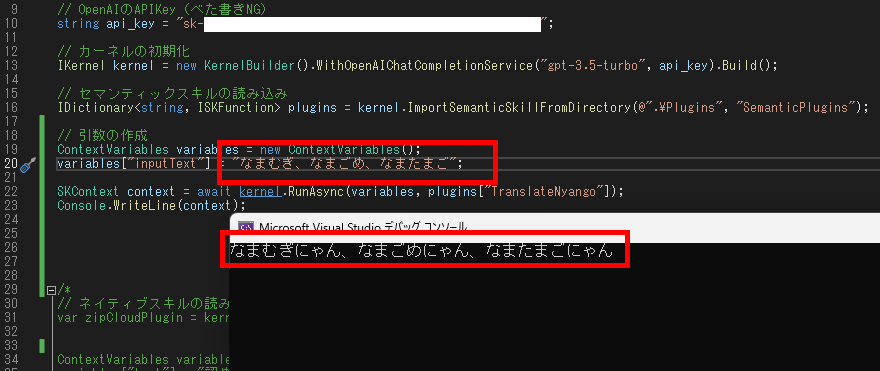
プロンプト自体にもう少し改良の余地があるけど、ひとまずこんな感じで動作確認はOK!
次回はNative Function(Native Skill)を作ってみる。
コメント
実行結果のapiが見えてますよ!
ご指摘いただきありがとうございます!!!
大変助かりましたm(T T)m